Stock markets are highly complex systems and cannot be easily predicted. The main objective of financial management of any firm is the maximization of the stakeholder’s wealth and intelligent estimates leading to accurate prediction of the stock market ensures that. This work presents the use of and comparison between Artificial Neural Networks, Recurrent Neural Networks i.e. Long-Short term Memory (LSTM), Convolution Neural Networks and Dilated Convolutions for the prediction of the future closing prices of stock market indices. It also proposes an architecture that is essentially a combination of the dilated convolution layers and LSTM that minimizes the error and surpasses the state of the art algorithms. The algorithm is tested on Dow Jones Industrial Index by using the data from December 2000 to December 2017. The paper explains the salient features of the above mentioned methodologies. The mean squared error (MSE) for various algorithms is recorded. As time passed and algorithms evolved we found that the MSE reduced and the algorithms better tracked the financial time series data. Thus the problem of stock market prediction has been efficiently addressed and is no longer an area that human beings are unsure of.
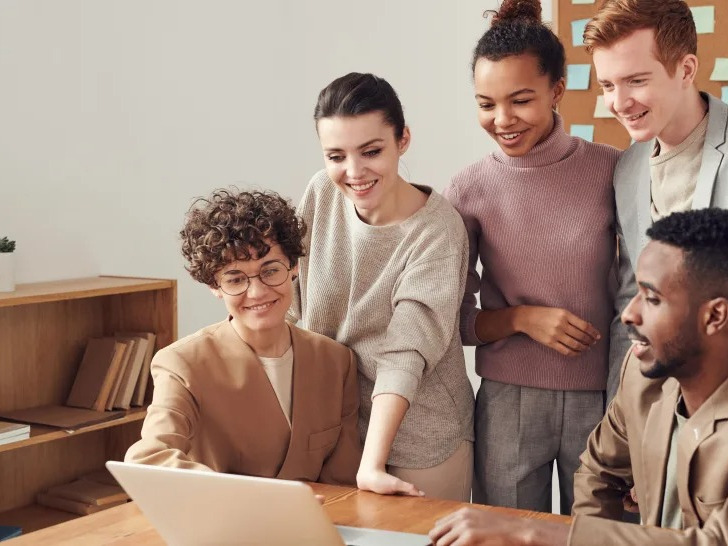
Bhumika Gupta, Monica Singhania, Arshiya Aggarwal. Financial time-series forecasting : from neural networks to dilated convolutions. AOM 2018 : 78th Annual Meeting of the Academy of Management, Aug 2018, Chicago, Illinois, United States.
- Date de publication
- 29 octobre 2019
- Catégories
- dans
- Auteur
- par Bhumika Gupta